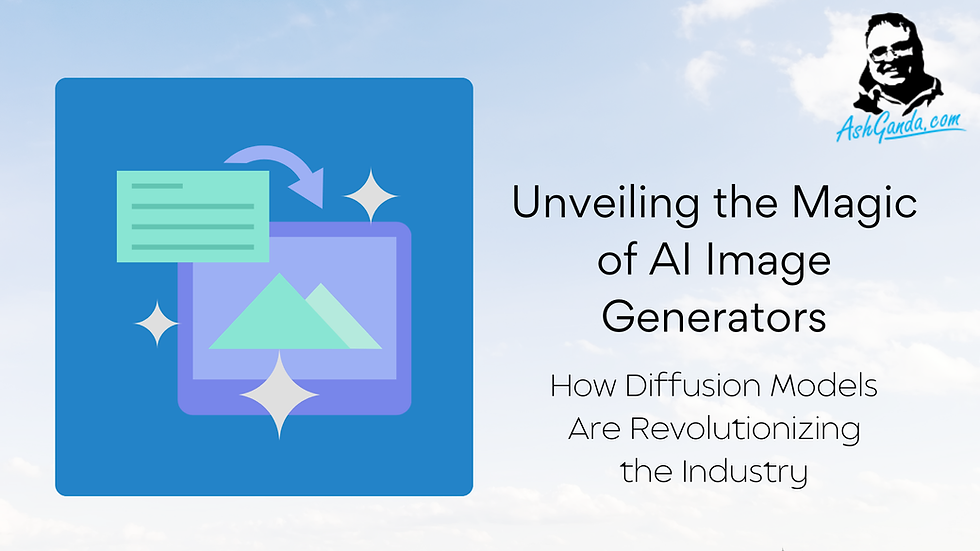
Introduction
Artificial intelligence has been making significant strides in the field of image generation, and it's hard to ignore the stunning images being produced by AI-powered tools. But how exactly do these AI image generators work? In this article, we'll delve into diffusion models and explore their role in revolutionizing the industry.
Traditional Methods: Generative Adversarial Networks (GANs)
Before exploring diffusion models, let's briefly examine the traditional method of using Generative Adversarial Networks (GANs) for image generation. GANs work by training a large neural network to produce an image by giving it random noise as input. This noise is then manipulated and refined until it produces an image that looks similar to the desired output, such as faces or landscapes. However, this method has its limitations, as it can often result in mode collapse where the network produces the same or similar images repeatedly.
The Power of Diffusion Models
Diffusion models simplify the process of image generation by breaking it down into smaller, iterative steps. This reduces the workload for the neural network, making it easier to generate high-quality images without any oddities or repetitions. But how exactly does this process work?
Understanding Stage Diffusion: The Magic AI Image Generator
Imagine you have an image of a rabbit and you add some noise to it. Then, you add some more noise to that image, and then some more, until eventually, you end up with a completely distorted image that looks like meaningless noise. The idea behind diffusion models is to reverse this process and train a neural network to undo this distortion.
The Role of Schedules
A schedule is essentially a set of instructions that dictate how much noise should be added at each step of the process. There are different strategies for creating schedules, but the general idea is to start with a small amount of noise and gradually increase it as the process continues. This approach allows for more precise control over the image generation process.
Benefits of Diffusion Models
One of the major benefits of using diffusion models is that it allows for easier training of the neural network. Unlike GANs, which can often result in mode collapse due to a lack of incentive for the network to create diverse images, diffusion models reduce the workload for the network, making it less likely to fall into this issue.Another advantage of diffusion models is their ability to generate high-resolution images. This is because they take an iterative approach, making small changes at each step instead of trying to create an image in one go. This can result in smoother and more detailed images.
Real-World Applications
AI image generators like DALL-E and stable diffusion use massive datasets to train their neural networks for specific tasks such as creating faces or landscapes, akin to magic. They then use diffusion models to generate images that are unique and diverse. This has led to some impressive results, such as the ability to generate images based on text prompts.
Ethical Considerations
However, there are also some ethical concerns surrounding the use of AI image generators. With the rise of "deepfakes," there are worries about these tools being used to create realistic but fake images and videos. This calls for ethical considerations and responsible use of AI image generators to avoid any potential harm.
Conclusion
Diffusion models have revolutionized the field of AI-powered image generation. By breaking down the process into smaller, iterative steps and using schedules to guide the amount of noise added, these models make it easier to train neural networks and generate high-quality images. As technology continues to advance, we can only imagine the endless possibilities and applications for these powerful tools.
Comments